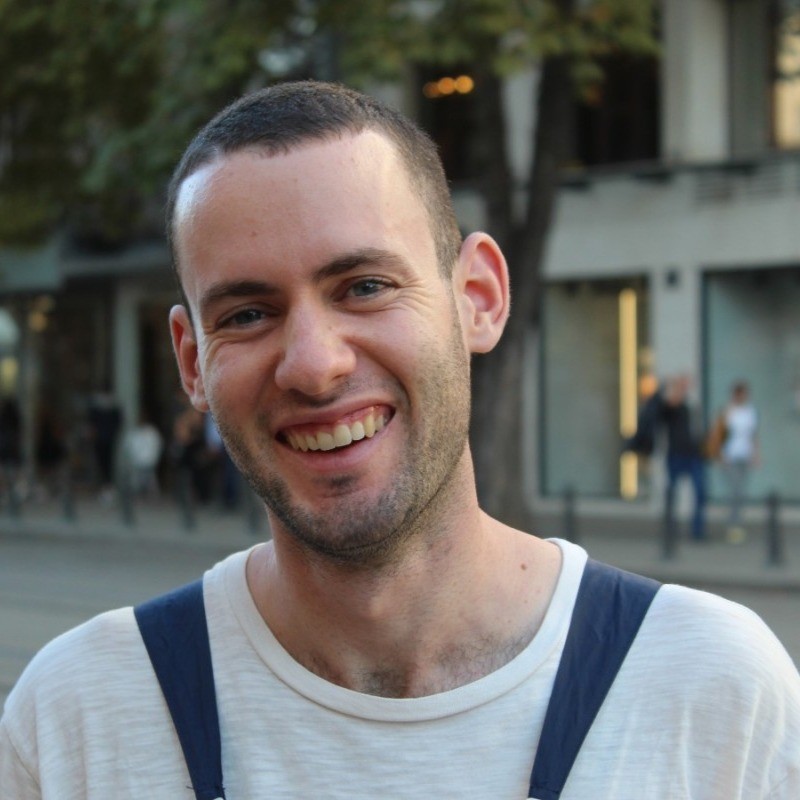
Matan Kleiner
I am a PhD candidate at the Electrical and Computer Engineering department of the Technion, under the supervission of Prof. Tomer Michaeli.
My research interests lie at the intersection of optics, computational imaging, computer vision and deep learning. I am exploring various theoretical aspects of optical computing, a promising paradigm that combines deep learning and photonics. Additionally, I am interested in the imaging pipeline, from accurately capturing objects in the physical world as images and videos to editing and manipulating them using vision foundation models.
Contact me at matan dot kleiner at campus dot technion dot ac dot il
.
Publications
Matan Kleiner, Lior Michaeli, Tomer Michaeli
Diffractive neural networks are a promising framework for optical computing. In this work we illustrate that, as opposed to imaging systems, in diffractive networks the degree of spatial coherence has a dramatic effect. We show that when the spatial coherence length on the object is comparable to the minimal feature size preserved by the optical system, neither the incoherent nor the coherent extremes serve as acceptable approximations. Following this observation, we propose a general framework for training diffractive networks for any specified degree of spatial and temporal coherence. Our findings serve as a steppingstone toward adopting all-optical neural networks in real-world applications, leveraging nothing but natural light. Presented as an oral presentation at CLEO 2024.
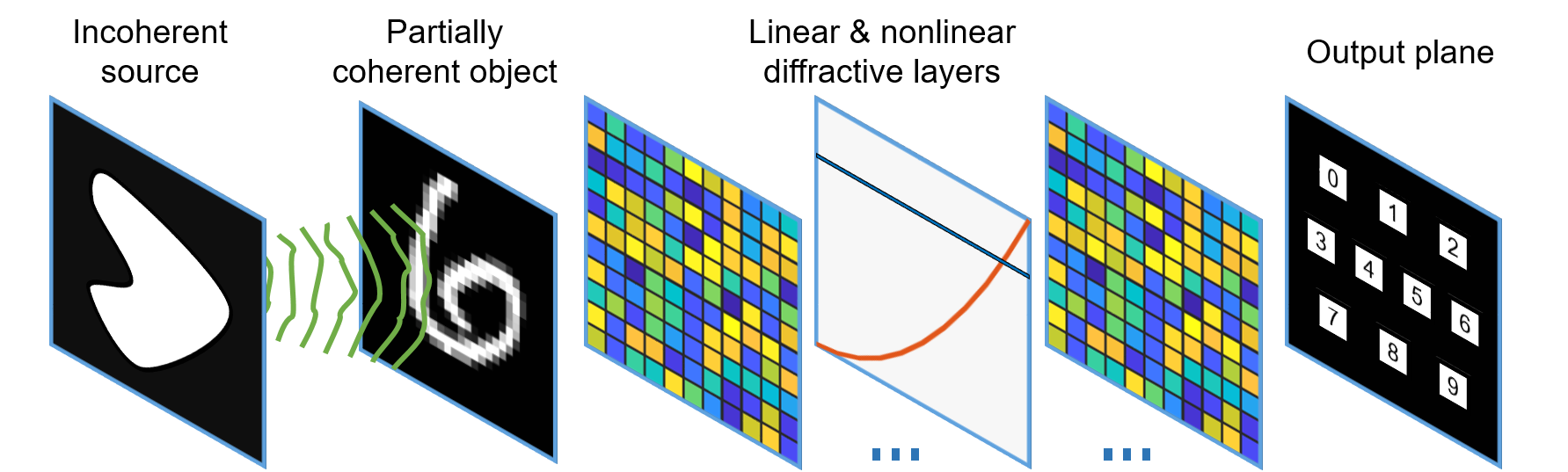
FlowEdit: Inversion-Free Text-Based Editing Using Pre-Trained Flow Models
Vladimir Kulikov, Matan Kleiner, Inbar Huberman-Spiegelglas, Tomer Michaeli
We introduce FlowEdit, a text-based editing method for pre-trained T2I flow models, which is inversion-free, optimization-free and model agnostic. Our method constructs an ODE that directly maps between the source and target distributions (corresponding to the source and target text prompts) and achieves a lower transport cost than the inversion approach. This leads to state-of-the-art results, as we illustrate with Stable Diffusion 3 and FLUX.
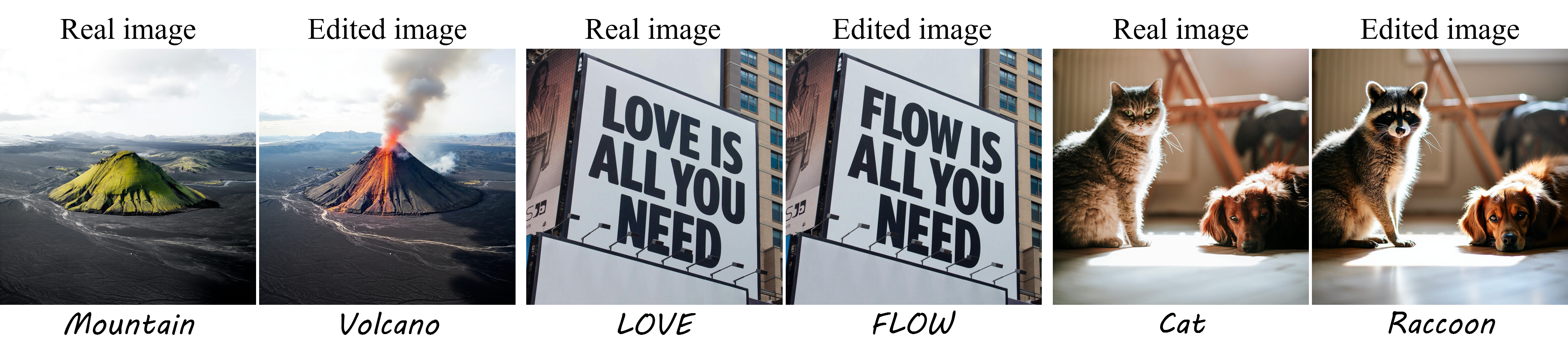
Slicedit: Zero-Shot Video Editing With Text-to-Image Diffusion Models Using Spatio-Temporal Slices
Nathaniel Cohen*, Vladimir Kulikov*, Matan Kleiner*, Inbar Huberman-Spiegelglas, Tomer Michaeli
We present Slicedit, a method for text-based video editing that utilizes a pre-trained T2I diffusion model and spatiotemporal slices. Spatiotemporal slices of natural videos exhibit similar characteristics to natural images. Therefore, the same T2I diffusion model that is normally used only as a prior on video frames can also serve as a strong prior for enhancing temporal consistency by applying it on spatiotemporal slices. Slicedit generates videos that retain the structure and motion of the original video while adhering to the target text. It edit a wide range of real-world videos, including videos with strong nonrigid motion and occlusions. Presented as an oral presentation at the CVG workshop @ ICML 2024.
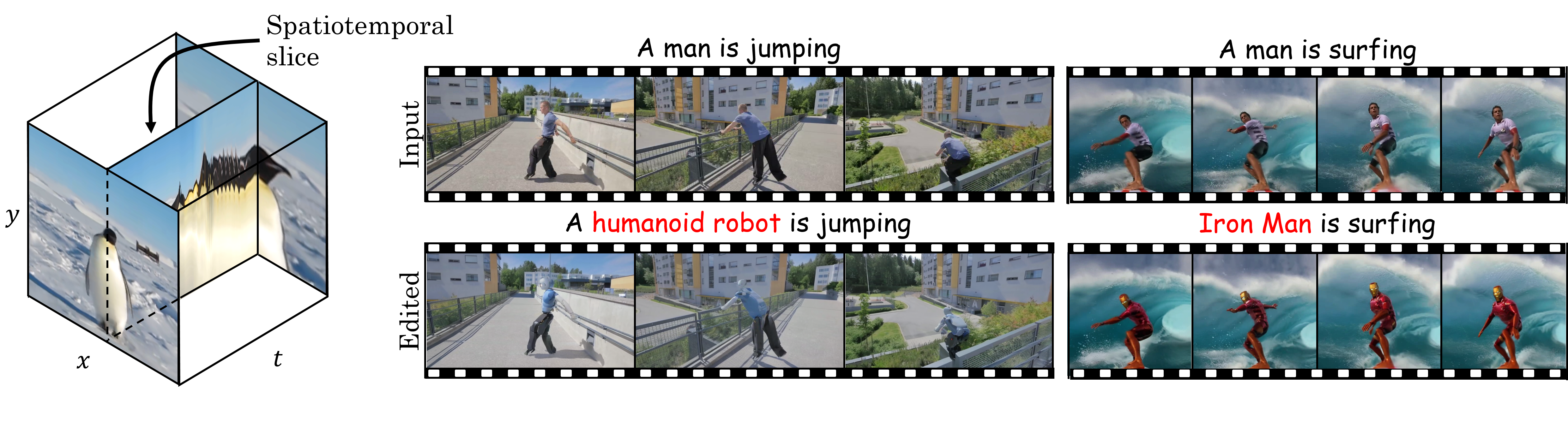
Vladimir Kulikov, Shahar Yadin*, Matan Kleiner*, Tomer Michaeli
We introduce a framework for training a DDM on a single image, which we coin SinDDM. SinDDM learns the internal statistics of the training image by using a multi-scale diffusion process and generate samples of arbitrary dimensions, in a coarse-to-fine manner. SinDDM generates diverse high-quality samples and it can be easily guided by external supervision, such as CLIP model.